Google AutoML
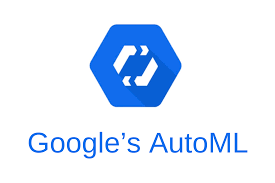
The Google AutoML: Revolutionizing Machine Learning with Automation:
Google AutoML: In recent years, the field of artificial intelligence (AI) and machine learning (ML) has rapidly evolved, enabling businesses and developers to harness the power of data like never before. However, the complexity of traditional machine learning processes often poses a significant barrier to entry, particularly for those without deep technical expertise. Enter Google AutoML, a suite of machine learning products designed to simplify the process of building and deploying custom machine learning models. By leveraging Google’s state-of-the-art technologies, AutoML empowers users to create powerful models without the need for extensive programming or data science skills.
In this article, we will explore the key features of Google AutoML, its benefits, and how it is transforming the landscape of machine learning for businesses and developers alike.
What is Google AutoML?
Google AutoML is part of Google Cloud’s machine learning offerings and provides a set of tools and services that automate the process of model development. The primary goal of AutoML is to make machine learning accessible to a broader audience, allowing users with limited ML expertise to train high-quality models tailored to their specific needs.
AutoML encompasses a range of services, including:
- AutoML Vision: For image classification and object detection.
- AutoML Natural Language: For text classification, sentiment analysis, and entity extraction.
- AutoML Tables: For structured data and tabular data analysis.
- AutoML Video Intelligence: For video analysis, including object detection and tracking.
- AutoML Translation: For building custom translation models.
Each of these services uses advanced machine learning techniques, including neural architecture search and transfer learning, to automatically optimize model performance based on user-defined criteria and datasets.
Key Features of Google AutoML:
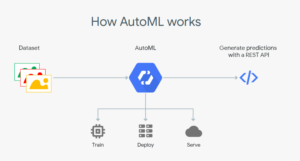
-
User-Friendly Interface
- One of the standout features of Google AutoML is its intuitive user interface. The platform is designed to be accessible to users with varying levels of technical expertise. By providing a straightforward workflow, AutoML allows users to upload their datasets, define their objectives, and start training models without needing extensive coding knowledge. The platform guides users through each step, making it easy to manage the entire machine learning lifecycle.
-
Automated Model Training and Optimization
- Google AutoML automates the entire process of model training, from data preprocessing to hyperparameter tuning. Users can simply upload their datasets, and AutoML takes care of selecting the appropriate algorithms, optimizing them, and training the models. This automation significantly reduces the time and effort required to develop machine learning models, allowing users to focus on their specific applications and use cases.
-
Transfer Learning
- AutoML leverages transfer learning, a technique that utilizes pre-trained models on large datasets to improve the performance of models on smaller, domain-specific datasets. This is particularly useful for users who may not have access to large volumes of labeled data, as it enables them to achieve high accuracy with limited training data. By fine-tuning existing models, users can quickly adapt them to their specific tasks, whether it be image classification or natural language processing.
-
Neural Architecture Search
- Google AutoML employs advanced techniques such as neural architecture search (NAS) to automatically discover and design optimal neural network architectures.
-
Integration with Google Cloud
- Google AutoML is tightly integrated with Google Cloud Platform (GCP), enabling seamless access to other cloud services and tools. The integration also allows for easy scaling, so users can handle varying workloads without worrying about infrastructure limitations.
-
Model Evaluation and Insights
- After training, Google AutoML provides detailed evaluation metrics and insights into model performance. Users can assess various performance metrics, such as accuracy, precision, recall, and F1 score, helping them understand how well their models are performing. The platform also offers visualizations and confusion matrices that help users diagnose issues and make informed decisions about model improvements.
-
Custom Model Deployment
- Once a model has been trained and evaluated, Google AutoML simplifies the deployment process. Users can easily deploy their models as REST APIs, making them accessible for real-time predictions. This feature enables businesses to integrate machine learning capabilities into their applications without the need for complex deployment processes. Additionally, Google AutoML supports batch predictions for processing large datasets efficiently.
-
Collaboration and Versioning
- Google AutoML allows teams to collaborate effectively by providing features for sharing projects, datasets, and models. Users can work together in real-time, enabling cross-functional teams to leverage each other’s expertise in developing and refining machine learning solutions. The platform also supports versioning, allowing users to track changes, revert to previous versions, and manage their models more efficiently.
Benefits of Google AutoML:
- Accessibility
One of the primary benefits of Google AutoML is its accessibility. By simplifying the machine learning process, AutoML enables users without extensive technical expertise to develop custom models. This democratization of machine learning empowers a broader audience, including small businesses and non-technical stakeholders, to harness the power of AI. - Efficiency
Google AutoML significantly reduces the time and effort required to develop machine learning models. Automation of model training and optimization frees users from manual tuning, allowing them to focus on business outcomes and application-specific goals. This efficiency is especially valuable in fast-paced environments where quick iterations are essential. - Cost-Effectiveness
By leveraging cloud infrastructure and automating many aspects of model development, Google AutoML can be a cost-effective solution for businesses. Users can save on the costs associated with hiring specialized data scientists and engineers, allowing them to allocate resources more effectively across their organization. - Scalability
As businesses grow, their data needs often increase. This scalability ensures that organizations can continue to leverage machine learning capabilities without being hindered by infrastructure limitations. - Continuous Improvement
Google AutoML supports ongoing model improvement through features like retraining and continuous learning. As new data becomes available, users can update their models to ensure they remain accurate and relevant. This continuous improvement process is crucial for maintaining competitive advantages in rapidly changing markets.
Use Cases for Google AutoML:
Google AutoML has been applied across various industries and use cases, showcasing its versatility and effectiveness:
- Retail: Retailers use AutoML for demand forecasting, inventory management, and customer segmentation. By analyzing historical sales data, models can predict future demand, optimize stock levels, and improve customer targeting strategies.
- Healthcare: In healthcare, Google AutoML is used for medical image analysis, patient outcome prediction, and drug discovery. For instance, AutoML Vision can assist radiologists in identifying abnormalities in X-rays and MRIs, improving diagnostic accuracy.
- Finance: Financial institutions leverage AutoML for fraud detection, risk assessment, and customer service automation. By analyzing transaction patterns, models can identify potentially fraudulent activities and enhance security measures.
- Manufacturing: Manufacturers utilize AutoML for predictive maintenance, quality control, and supply chain optimization. By monitoring equipment performance, models can predict failures before they occur, reducing downtime and maintenance costs.
- Marketing: Marketers employ AutoML for personalized recommendations, sentiment analysis, and campaign optimization. By analyzing customer behavior and preferences, businesses can tailor their marketing strategies to improve engagement and conversion rates.
Conclusion:
Google AutoML is transforming the landscape of machine learning by making it accessible, efficient, and scalable. By automating the complex processes involved in model development, AutoML empowers users with limited technical expertise to create custom models that meet their specific needs. The integration of advanced techniques like transfer learning and neural architecture search ensures that users benefit from state-of-the-art performance without the steep learning curve traditionally associated with machine learning.